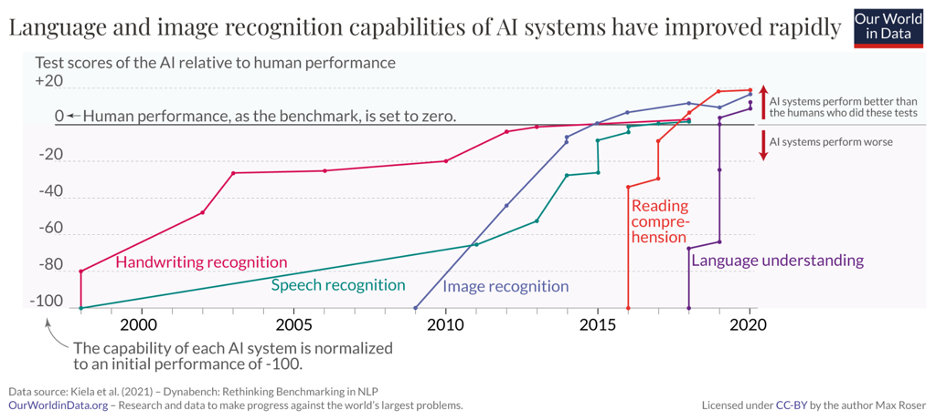
The impact of machine learning (ML) on fields like natural language processing and image recognition is well documented (e.g. https://ourworldindata.org/brief-history-of-ai). To make that impact, researchers developed models which utilized large amounts of annotated data to unearth correlations that were simply not possible using other approaches. In fact, the ability to access and process annotated data has been directly correlated with the increasing speed of ML maturity.
Using a data-driven approach via ML to improve the state-of-art in wireless design is one of the founding principles behind Aira Technologies. In wireless networks, huge volumes of data are already generated and processed in both the control and data planes, most of which is annotated. Tapping into the potential of ML for wireless design will depend on the ability to curate and present the wireless data as part of ML training. This is where we, at Aira Technologies, are creating value.
We have applied ML to hard problems in wireless with great success as evidenced by our announcements in MWC 2023. Our Channel Prediction xApp resulted in 2X improvement in throughput and our intelligent Energy Management rApp resulted in 25% reduction in RAN energy consumption. These sorts of improvements in wireless performance are only possible by applying the data-driven approaches of AI/ML.
We believe ML is here to stay as an integral part of wireless design.
- ML makes wireless design more flexible
At Aira, we believe that given enough time, a team of competent system engineers can uncover a near-optimal solution to most static problems. However, real-world wireless networks are not static. RF environments change, RF impairments appear asynchronously, user behavior varies over time, availability of signals change across platforms, and even standards change. In the face of constant change, a data-driven, ML-powered design is more likely to stay true to the current context than a model-based, algorithmic design.
- ML increases the speed of wireless design
In this fast changing industry, time to market matters. The amount of time and effort it takes to retrain a ML network to include a newly available signal, or to adapt to changes in the format of signals, or to deal with the absence of a signal, is miniscule. This is especially true when compared to re-deriving the optimal signal processing for the same set of changes using a traditional algorithmic approach.
- ML focuses wireless design on what matters most
The RAN stack uses modules from many fields. Estimation theory, information theory, statistics, coding theory, DSP, control systems, queuing theory and more. Each field has a slightly different nomenclature, slightly different terminology and slightly different set assumptions. We find that using ML in key functional blocks across the stack makes the designs much more uniform and modular.
We do not use ML just for the sake of it, and in fact, as information theorists, we believe that some blocks in L1 have near-optimal design. We do not seek to reinvent the wheel when the benefit is just not there. However, some implementations in L1 are not yet optimal and there are many blocks in L2 and above that have no real framework for optimality. A data-driven approach using ML thrives in such cases.
With our Channel Prediction xApp and the Energy Management rApp, Aira has just scratched the surface of what is possible with ML in wireless. Aira has been working on some of the other key areas of applicability of ML in Cellular: Physical Layer, MAC Layer and Core Network Layer. Each contains their own challenges and opportunities for ML.
Learn more about Aira’s approach to applying AI/ML to wireless.
Learn more about radical changes to network architecture that are being enabled by Open-RAN